When a systemic shock hits the financial system, it causes extensive damage that requires a great deal of resources to clear up. In a perfect world, it would therefore be much more beneficial for governments, businesses and consumers if the build-up of risk were identified early enough to enable policymakers and market participants to respond.
Significant effort is currently being invested in empirical techniques to identify systemic risk. However, some of this work, which is based on studying directly observable outcomes in financial markets, may not identify systemic risk until it is too late to take action.
The SRC has therefore embarked on a number of research projects, some in close collaboration with our data partner IHS Markit, which aim to study the underlying risk factors leading to systemic risk and thus empirically capture endogenous risk.
This will involve a combination of statistical analysis, theoretical modelling and practical description of the political system. Within this, the SRC is actively investigating four main empirical research strands.
1. Computer-based trading
Computer-based trading (CBT) – and in particular the special category of high frequency trading (HFT) – is taking over much of the trading in financial markets. These trading activities can alter the characteristics and the dynamics that, in turn, feed into the values and price signals guiding investment behaviour.
This phenomenon has raised concerns among investors and market commentators. Some highlight the algorithms that follow simple rules at lightning speed, which means that human traders are bound to lag behind. Another concern is that robot computers follow these rules mechanically, without sense of discretion.
Case example: 2010 “flash crash”
The US stock market experienced a very sudden crash on 6 May 2010, plummeting almost 10% in a matter of minutes and recovering just as quickly. A joint report by the Securities and Exchange Commission and the Commodity Futures Trading Commission identified a single institution selling a large number of E-Mini S&P 500s, a futures contract, which prompted HFTs to start selling aggressively. The crisis in the futures market quickly spilled over into equities. Computer systems used by many HFTs detected the large volumes and extreme price movements, consequently scaling back their trading or withdrew from the markets altogether, creating a liquidity drought. The crisis was eventually halted when the Chicago Mercantile Exchange implemented automatic stabilisers.
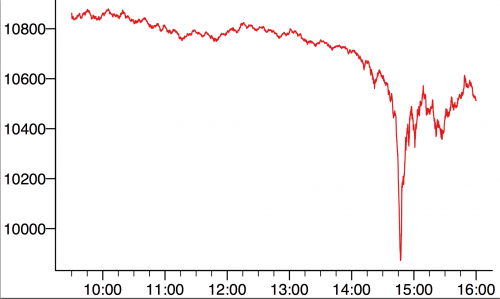
The SRC believes these concerns make the potential systemic risks within these trading systems a promising and fast-moving area for researchers to analyse. A preliminary collection of such systemic events can be found in the UK Foresight Report on the Future of Computer Trading, to which SRC researchers contributed. In order to help the SRC study HFT environments, the Computer Science Department of University College London is a co-investigator.
2. Market-based data and risk forecast models
The most obvious way to empirically model systemic risk is to employ market-based data. However, the failure of many of the risk forecast models used by the financial industry and authorities to identify the build-up to the global financial crisis suggests that model risk is a key area for research.
The SRC believes that there is a question mark over the suitability of direct indicators of risk, such as the VIX volatility index or CDS spreads, and off-the-shelf market risk methods, such as value at risk (VaR). Analysis of endogenous risk suggests such widely available market-based indicators react only after a crisis is underway, not before.
3. Model risk of bank pricing risk
Financial institutions which use complex financial instruments traded over-the-counter face the challenge of how to evaluate the accuracy of their internal pricing models.
One way they address this problem is by sending their prices for a range of complex instruments to a commercial service run by IHS Markit called Markit Totem, which provides them with an indication of where their prices are relative to their competitors.
Markit is providing the Totem database to the SRC, enabling its researchers to address fundamental questions of model risk and model reliability, as well as liquidity and uncertainty, in complex derivatives.
4. Securities lending
Securities lending refers to the practice of lenders transferring securities to borrowers, who commit to returning securities of the same type, quantity and quality at a later date.
Markit is providing the entire securities lending database, containing in excess of 100,000 observations a day, to the SRC. This enables researchers to address fundamental questions on the nature of liquidity and how it is affected by stress situations, broken down by different industry and country. This is a unique database and one to which few, if any, other academic researchers have complete access.
Aim of this research strand
A key part of the mission of the SRC is to analyse and gain a greater understanding of the empirical aspects of how systemic risks build up in these four different areas for which we have access to data. We aim to build tools that will enable regulators and market participants to identify and measure systemic risks before they crystallise, and thus give time for them to react.